Data-driven triage for specialty insurers and its value to underwriters
2 minutes
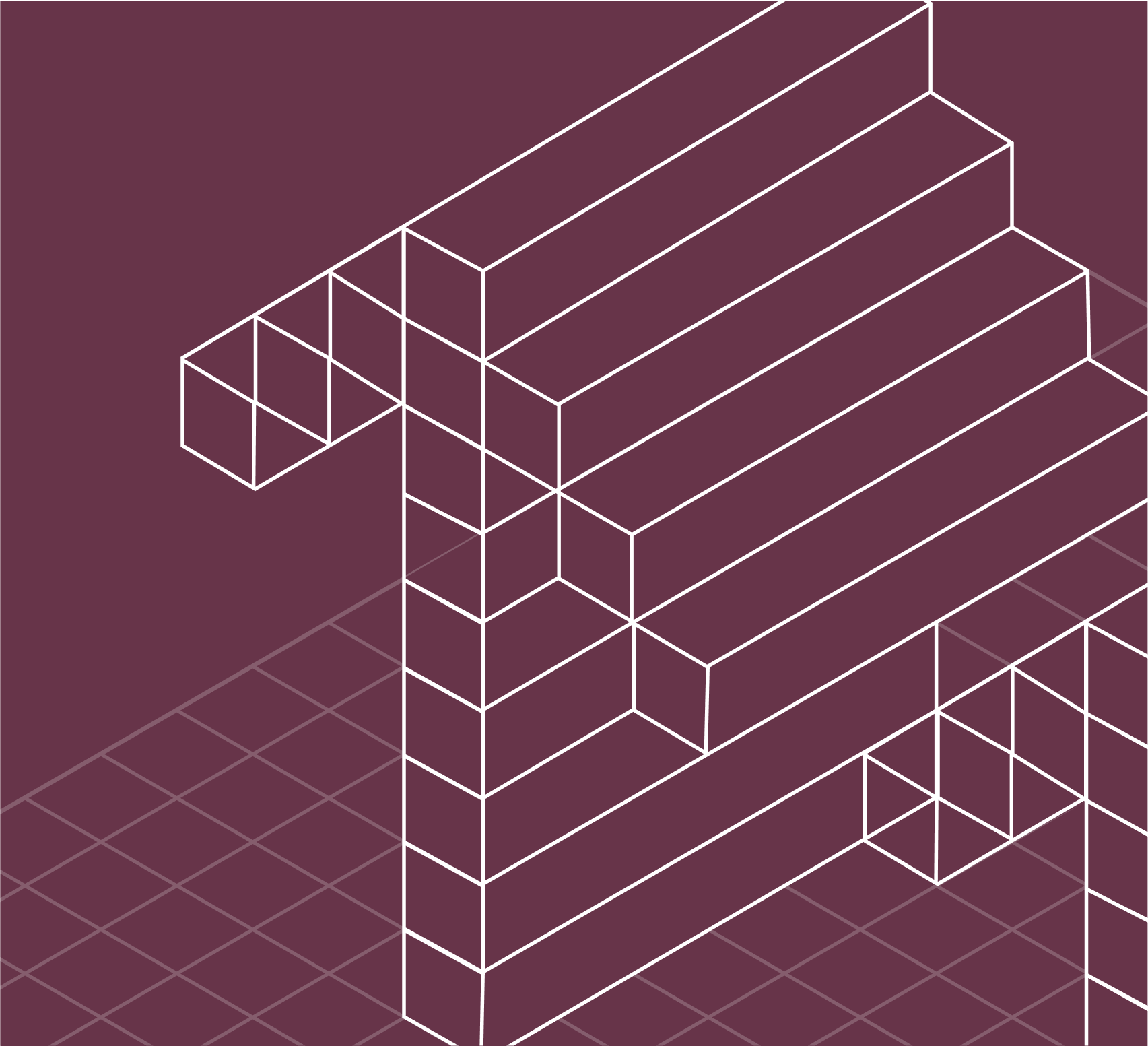
See how underwriters are leveraging data-driven triaging to save time and make smarter business decisions more efficiently.
As an underwriter, especially in a high-volume business, it is possible to receive many requests from brokers and struggle to find enough hours in the day to review, model and price each one. One of the challenges you face is that until you explore each request and apply some, or all, of your processes, you have no idea how much risk you're taking on and cannot decide if it makes commercial sense to underwrite the policy.
Now, imagine you could automate part of this work, effectively implementing a triage process that categorises the risk of each request and guides you on where you need to focus your attention. What difference would it make if requests that fall outside of your underwriting guidelines for risk could be automatically rejected without your need to review? What if you could be alerted to areas of concern on other requests, and see which of these will only require minimal review?
Suddenly, as an underwriter, you gain valuable time back, and you can focus on applying your skills and expertise in the areas where you can deliver the most value to your business.
What is data-driven triage?
Data-driven triage is a way of performing fast-track risk scoring, applying previous experience to new risks, informing the current underwriter pipeline and identifying those likely to be within appetite.
In its simplest form, this is applying a rule. It is taking parameters of interest and either calculating a weighting or using simple decision logic. For example, a specific property type may be outside of your appetite and, as such, can be quickly rejected without underwriter time being consumed.
Data-driven triage becomes even more of a game-changer when you apply machine learning to the process, using a wider set of historic data and allowing algorithms to make predictions. This is based on a broader set of parameters to make smarter predictions and can present the underwriter with pre-populated guidance on each risk.
This guidance can be as simple as a red, amber, or green categorisation – outside of appetite, needs review or fully aligned with appetite – or extended to more sophisticated metrics and risk scores on multiple factors to generate a weighted percentage. The amount of data that is available to us is sometimes broader than we realise; having access to past information is one thing, but there are several additional data sources that can be used to automatically augment existing datasets and help ensure a more accurate, data-driven approach.
The value to specialty underwriters and insurers
Think about Netflix or Amazon, where machine learning is applied to personalise recommendations to you about what to watch or buy. In a similar manner, data-driven triage can effectively recommend which risks or renewals you might like.
By providing automated guidance, underwriters will know where they should focus their effort and spend more time adding value, generating more profitable business. When combined with the data-driven approach and other methods to digitise the pricing process, such as Optical Character Recognition, underwriters have easier access to the information they need to make even better decisions.
Is data-driven triage a future or a reality?
At hx, we are already helping customers build their data-driven triaging. With hx Renew, the data for historical policies is structured, enabling the rules to be built and to automate the triage process.
Given the flexible nature of Renew and our use of open source Python, underwriters and developers can set up a machine learning pipeline in Renew, leveraging a broad range of leading machine learning algorithms that plug straight into our platform, such as XGBoost or TensorFlow. This allows for the rapid creation of automatic triaging, and, on an ongoing basis, a machine learning algorithm can continuously learn from written policies in a positive feedback loop to improve its own performance and make better predictions.
As pointed out in McKinsey’s Insurance 2030 – The impact of AI on the future of insurance, leveraging data and applying machine learning will enable insurers to enhance decision-making, drive greater productivity and ultimately write more profitable business. At hx, we believe insurers can gain such benefits much sooner.